Recently, there’s been a lot of interesting activity in the field generative AI for science from large companies such as Google, Meta and Microsoft.
Creating new materials from scratch is difficult, since materials involve complex interactions that are difficult to simulate, or a fair amount of luck in experiments (serendipity is scientists’s most terrifying friend)
Thus most of these efforts aim to discover new material by accelerating simulations using machine learning. But recent advances (such as LLM, e.g., ChatGPT) have shown that you can use AI to make coherent sentences instead of a word soup. But the same way cooking is not just about putting ingredient together all at once but carefully preparing them, making a new material involves important intermediate steps. And new approaches can be used create new materials.
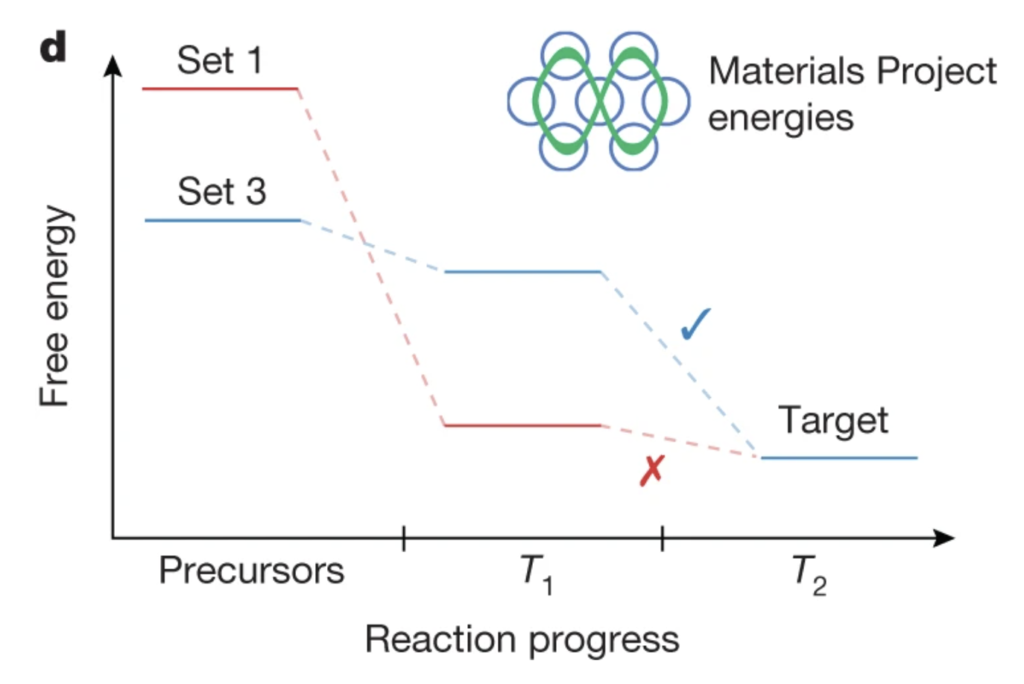
The various steps of making a new material (from Szymanski et al.)
Last month, Google in collaboration with Berkeley Lab announced that their DeepMind’s Gnome project had discovered a lot of new structures: Google DeepMind Adds Nearly 400,000 New Compounds to Berkeley Lab’s Materials Project. They managed to actually make and analyze some of those new materials ; that is quite a tour de force, and while there’s some interesting pushback on the claims, it’s still pretty cool!
In September, I invited Meta’s Open Catalyst at Berkeley Lab (here’s the event description and the recording – accessible to lab employees only)
Meanwhile, Microsoft is collaborating with Pacific Northwest National Laboratory on similar topics
And it’s not limited to US tech firms: Why Is TikTok Parent ByteDance Moving Into Biology, Chemistry And Drug Discovery? – Forbes
Meanwhile, the research infrastructure has it gears moving; it seems that DeepMind’s AlphaFold is already routinely used at the lab to dream up new protein structures. I wonder where this will go!
Prediction is very difficult, especially if it’s about the future– Niels Bohr
Thinkpieces blending chips and AI in full bloom:
We need a moonshot for computing – Brady Helwig and PJ Maykish, Technology Review
The Shadow of Bell Labs
I want to resurface an interesting thread by my former colleague Ilan Gur:
Interested in bringing back Bell Labs?
- Some thoughts on why it’s not possible, and what we should do instead:
At peak, Bell Labs was about $2B/yr in today’s USD. Split 30% fundamental research, 10% translation, 60% systems engineering. (straight from the source) - For perspective, we’re talking about a corporate R&D lab with a fundamental research arm twice the size but same caliber as Cal Tech alongside a systems engineering team roughly the same size/caliber of Space X (guessing), with a translation team >10x the size of Otherlab. So please just quit with “X is the modern day Bell Labs”
- Next: bear in mind that after WWII, academic research was small & ed focused (mainly philanthropy funded). So Bell Labs could control a significant portion of the fundamental research pipeline. That’s no longer possible with U.S. gov alone now funding ~$50+B/yr to univs and FFRDCs. For that reason alone, it’s not possible to recreate Bell Labs today.
- That said, what we can (and should) recreate is the tight connection that existed in those days b/w fundamental research & application. At Bell Labs, science & application lived under one roof, which is all too rare today…for reasons I dive into here: How the US lost its way on innovation – Ilan Gur, Technology Review (2020)
So how to bring back the mojo of Bell Labs today? Follow the trends:
– big corps no longer science powerhouses
– fundamental research pipeline now huge & distributed
– talent no longer needs big institutions for knowledge, networks, tools
Biontech and ModernaThere’s just one problem: we only get startups around people/ideas that VCs are willing to bet on at any given moment.Isn’t it weird that we’re ok depending on VCs making crazy early out-of-the-money option bets in order for top talent to translate scientific research into products in an aggressive way? The first few years of a deep science startup are basically focused, aggressive applied R&D. It’s cool VCs can sometimes cover that, but it’s nowhere near enough. What about people not plugged into VC, or ideas that need more time/resources or don’t fit the latest hype cycle? /12This is where we need government. Bell Labs is remembered as a great INDUSTRIAL research lab, but only existed thx to gov funding (via a gov-sanctioned monopoly). Gov $ is the *only* way to support applied R&D at the scope & scale needed to serve society on #climate & beyond.How can the U.S. invest toward Bell Labs outcomes? Start by modernizing science & industrial policy, recognizing that the U.S. advantage in [science x entrepreneurship] is enormous & still largely untapped in its potential to create opportunity, prosperity, & a better future.Bring back Bell Labs? Nah… But maybe we can do even better.Original thread:https://twitter.com/ilangur/status/1353215030196531201
== end of thread =
Case in point, most efforts by large company having too much disposable income, such as Meta “building 8” and Google “X”, failed in their efforts, despite formidable resources:- TECH How Facebook failed to break into hardware: The untold story of Building 8, CNBC (2019)
- Alphabet’s Moonshot X Lab Cuts Staff, Turns to Outside Investors (Bloomberg, 2024)
It is quite obvious: making new technologies is very hard (it’s more than just throwing away money at a problem), and big corporation do not have the flexibility of a dozen startup: they need to know what the winning technology will be before even starting: an impossible task, given that the landscape can change quickly.